My Observations of the Current Data Science Industry and Career Advice - an SEA point of view
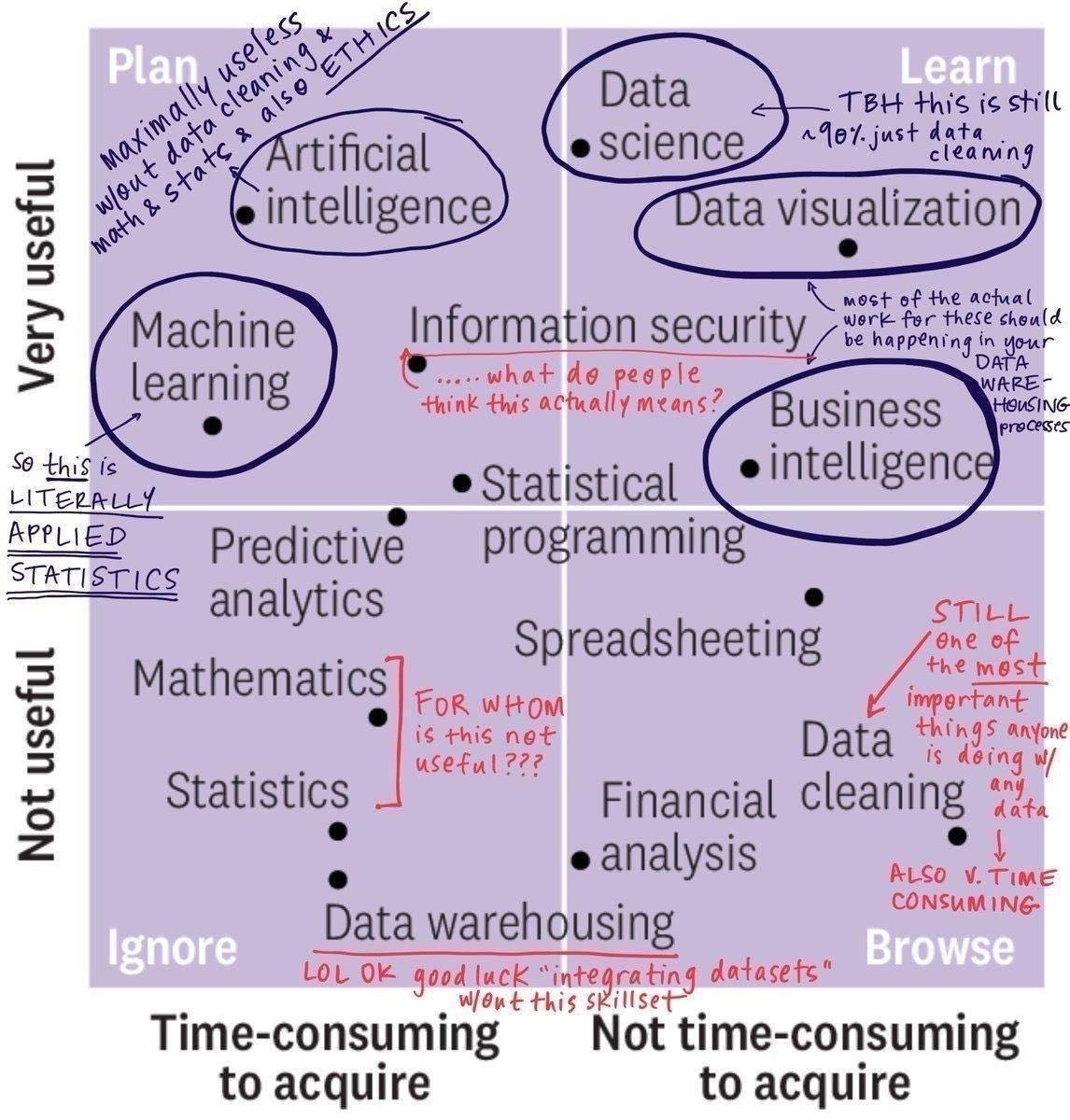
A short bio of myself. I am a 1 year old data scientist, based in Singapore. Without a computer science or a statistics degree, I spent 3 years pursuing a career of data science before I landed a full time DS job. In this blog, I’d like to share my understanding of the data science industry and give some advice to the friends who are keen to pursue a DS career. The opinions expressed here represent my own, and are given based on the experience of my work, social networking, as well as the books and articles I read.
1. The title of data scientist is undergoing an “inflation”.
Due to the increasing attention to AI and big data in recent years, more and more companies claim that they are adopting AI technology in their product, and are looking to hire data scientists. However, does a company require many data scientists, and are the “data scientists” really doing the actual data science work? The title of “scientist” is nice to hear, but the daily work of most data scientists is far from the actual scientists, e.g. the statisticians and physicists who work on the cutting-edge research topics at research institutes. Therefore, the first advice for the friends who are keen to pursue a DS career is that, don’t get fooled by the fancy title. It is necessary to understand the DS role and choose the job which best fit your interest and strength.
Understand the DS lifecycle and find your interest and strength
I was asked by a few friends about what data scientists usually do as their daily work. Known as the sexiest job of the 21st century, data scientist is in fact not an independent job but requires extensive collaboration in between various roles. It’s very helpful to gain a good understanding of the different roles in a typical DS lifecycle.
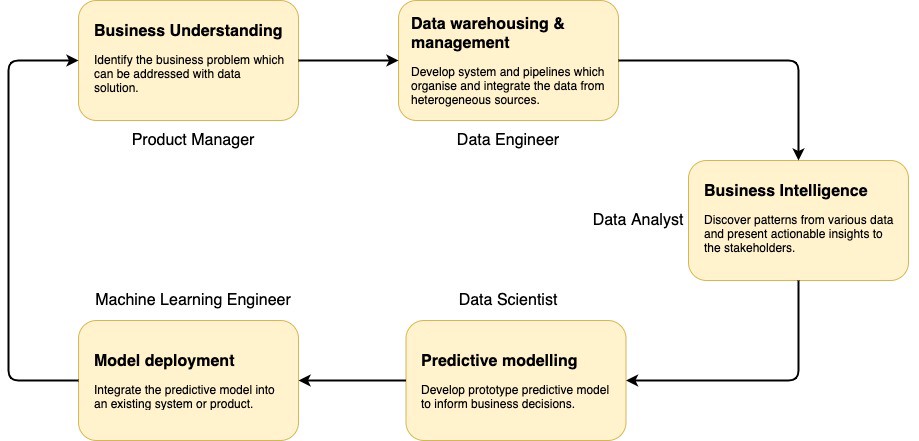
Figure 1 A typical data science life cycle
Figure 1 gives the common roles in a typical data science life cycle. In general, the main responsibility of data scientists is to develop machine learning models with appropriate algorithms and tools to address specific business problems such as fraud detection and demand forecast. At the downstream, machine learning engineers take the prototype models from notebook to production. All the above roles are indispensable in a DS project. At startup companies, it is possible that one person needs to undertake multiple roles.
Cassie Kozyrkov wrote an amazing blog, which gives a detailed introduction of various data related roles involved in different project phases.
2. The values of data scientists at different companies are measured by the business impacts they bring.
As one of the new favourites among various IT professionals, data scientists are expected to have a superior earning power. However, this is not true, at least in South East Asia. Figure 2 shows a comparison of average annual salary between data scientists and software engineers in Singapore and San Francisco.
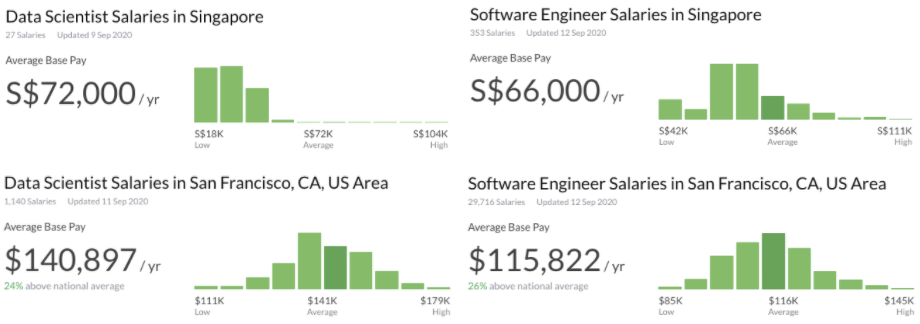
Figure 2 Salary comparison of data scientist vs. software engineer
The pay gap between data scientists and software engineers in Singapore is much smaller than that of San Francisco. This indicates that the DS industry in SEA is not mature yet. Most SMEs cannot appreciate the capability of the DS and ML technology. They are not aware of what can be achieved with the DS capability or how to build a DS workforce.
Another interpretation of the small pay gap is the limited business impact brought by the data scientists in SEA. In terms of the complexity of the work and the requirement of the tech skills, data scientists and software engineers could be similarly challenging. For most IT companies, developers are always the backbone of their tech team. Their product cannot survive without the contribution of the developers while their data scientists might be able to boost the profits by 5% in the ideal case. Can you get the difference here?
Hence, the second advice I’d like to give here is to evaluate the position and potential impact you can bring to the business as a data scientist, when you choose a DS job.
Evaluate the position and potential impact you can bring to the business as a data scientist, when choosing a DS job.
A few pointers you can ask yourself.
- Is DS the core technology for the company’s product?
- As a data scientist, will you be participating in the development of the product, or some system for the internal use?
- What kind of problems and projects will you be working on? Is it what you like?
There is no right or wrong, good or bad to the answers. It all depends on where your interest lies.
3. The research and experimental nature of DS makes it challenging but fascinating.
If you reach here, I hope you have not been discouraged from pursuing DS by the first two observations. DS becomes fascinating when you get to work with real world problems, e.g. user behaviour, marketing strategy, various factors which can affect the revenue. Data scientists feel the most fulfilled when the application they developed can predict the future decisions of the users and drive the growth of sales. This cannot be achieved without tedious data manipulation, extensive research, and repetitive experiments. When the business goals are met, all the hard work will be worth it.
In addition, DS requires more business thinking and creativity. How to frame the problem to make it solvable with existing data? What kind of features shall be used by the model? What metrics should be used to measure the performance? None of these questions are straightforward to answer. This is why the domain knowledge plays an important part of the DS work. This is also what differentiates data scientist from a developer.
Having known its pros and cons, if it is something you like, aim for it and make determined efforts. There are tons of material introducing how to prepare for a DS career. Here I will only give a quick summary.
- Prepare for the technical skills: math, programming, machine learning.
- Build up a portfolio of DS projects. Competitions and self-initiated projects are a good starting point.
- Read and talk to DS folks to understand how the technology is applied in different walks of industry.
This blog gives a practical guideline of self-learning DS. I used to get overwhelmed with various resources: online courses, books, competition platforms. The correct way is to find one good material for each topic and follow through it. Practice makes perfect.
Thanks for the read. I welcome feedback and constructive criticism and can be reached on LinkedIn.